Blog
Predictive analytics can often be seen (or sold) as a magic wand that will predict the future, and with the market potentially set to reach up to $63.3 Bn globally by 2032, it’s becoming increasingly difficult to know what’s actually worth the investment.
Now, skepticism is definitely not new when it comes to technology and data, and some of it is more than justified. However, there’s somewhat of a reputation of smaller businesses in particular being less tech savvy and therefore more reluctant to adopt new tools and ‘moving with the times. Realistically, this reluctance is often underpinned by smaller margins and fewer resources, financial or otherwise), not a lack of knowledge or ambition.
The majority of predictive AI models and projects, for want of a better word, fail. International organisations with a workforce of thousands and a turnover of billions have the luxury to test, fail and learn without putting the entire business at risk. Small businesses simply cannot risk their time, money and customer satisfaction only to realise their intuition is still more accurate than this new tool management has forced on them.
The barriers to AI adoption among SMEs are by far insurmountable, but in order to understand how to overcome them, let’s first look at why so many predictive analytics projects don’t succeed…
Data collection, quality, and use
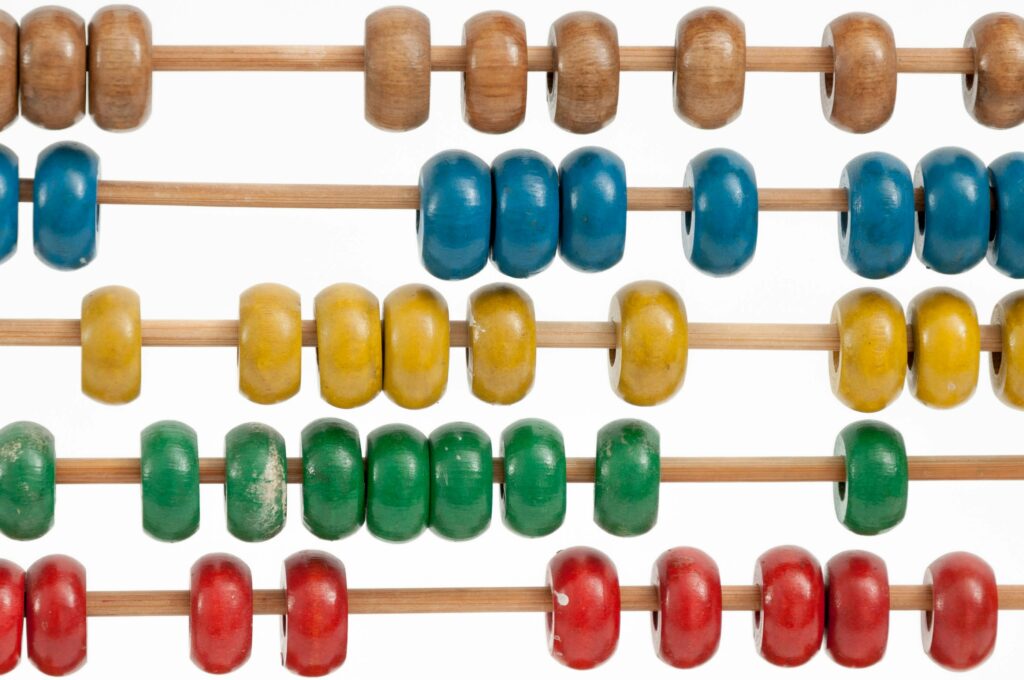
Unsurprisingly, when it comes to predictive data analytics, the data itself is pretty critical. It’s typically understood that the more data the better.
In order for models to make reliable estimates on what will happen, they first need to know what has happened in the past, why it has happened, and the role different factors play in those outcomes. To do this, and to do this as accurately as possible, it needs the right data. Not simply, lots of data, but the right data.
Yes, traditionally the best models required quantity and structure but we’re not in a position where predictive accuracy doesn’t have to be sacrificed when the data is small, full of gaps, and incomplete. But again, it comes down to having the right data for the model.
This could mean filling in gaps using external data sources or shifting entirely to a different type of machine learning algorithm. Not every model or technology is suited to everyone’s data, find (or build!) the right one for you.
Results. Results. Results.
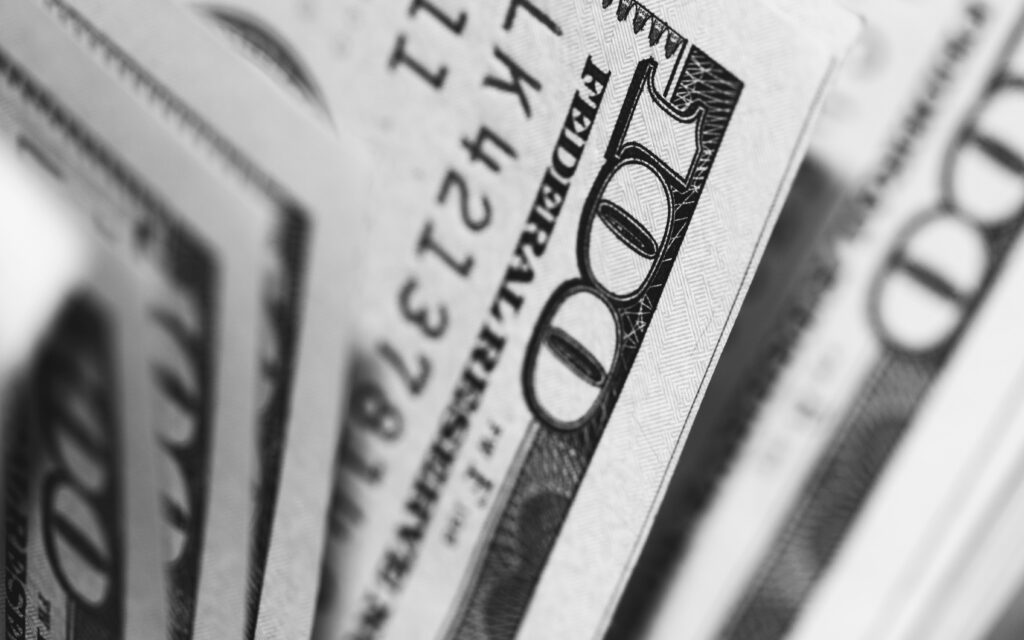
At the end of the day, it all comes down to results. Is the technology producing accurate (or accurate enough) predictions and insights that either increase revenue or efficiency?
Large companies will likely give it months until reviewing the performance of a new tool or technology. After all, the process to implement takes a long time when so many decision makers are involved.
Small business owners or managers on the other hand have to move quickly. Not just with setup, but if they don’t see a material difference within a few weeks, they might decide to cut their losses and stop.
In these situations, models/solutions need to ensure they can provide speed across every stage – implementation, scaling, insight generation, etc. – in order to demonstrate value as soon as possible.
Expectation vs Reality
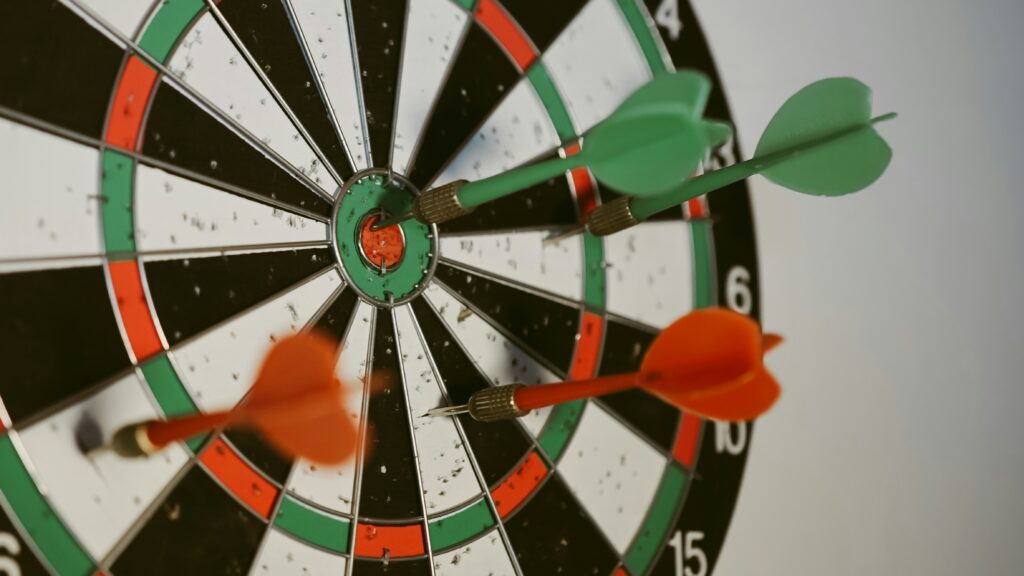
Sadly, there is no magic wand (yet!) that is 100% accurate and gives you everything you need with a single click. That’s why it’s so important to set clear, measurable, and realistic objectives… as you would with any project or campaign.
And part of this is understanding the potential limitations of the technology/models used. Whether building your own tool or using a third party solution, acknowledging that not even the most accurate and complex model can predict the future 100% of the time.
Blindly following whatever numbers come out may be tempting, but could have disastrous consequences. The value in having a data partner like TUBR is not simply in the predictions, but the ability to help you understand the different outputs within the context of your business, giving you the best chance of success.
Learn more about TUBR’s predictive technology here.